Artificial neural networks in the modeling and optimization of aspirin extended release tablets with Eudragit L 100 as matrix substance
Само за регистроване кориснике
2003
Аутори
Ibrić, Svetlana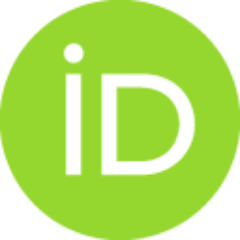
Jovanović, M
Đurić, Zorica
Parojčić, Jelena
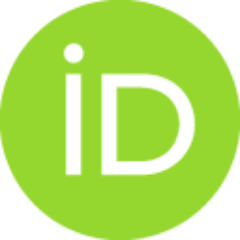
Petrović, Slobodan D.
Solomun, Ljiljana
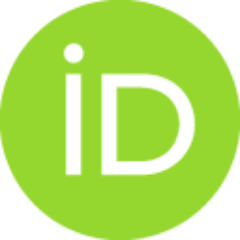
Stupar, Biljana
Чланак у часопису (Објављена верзија)
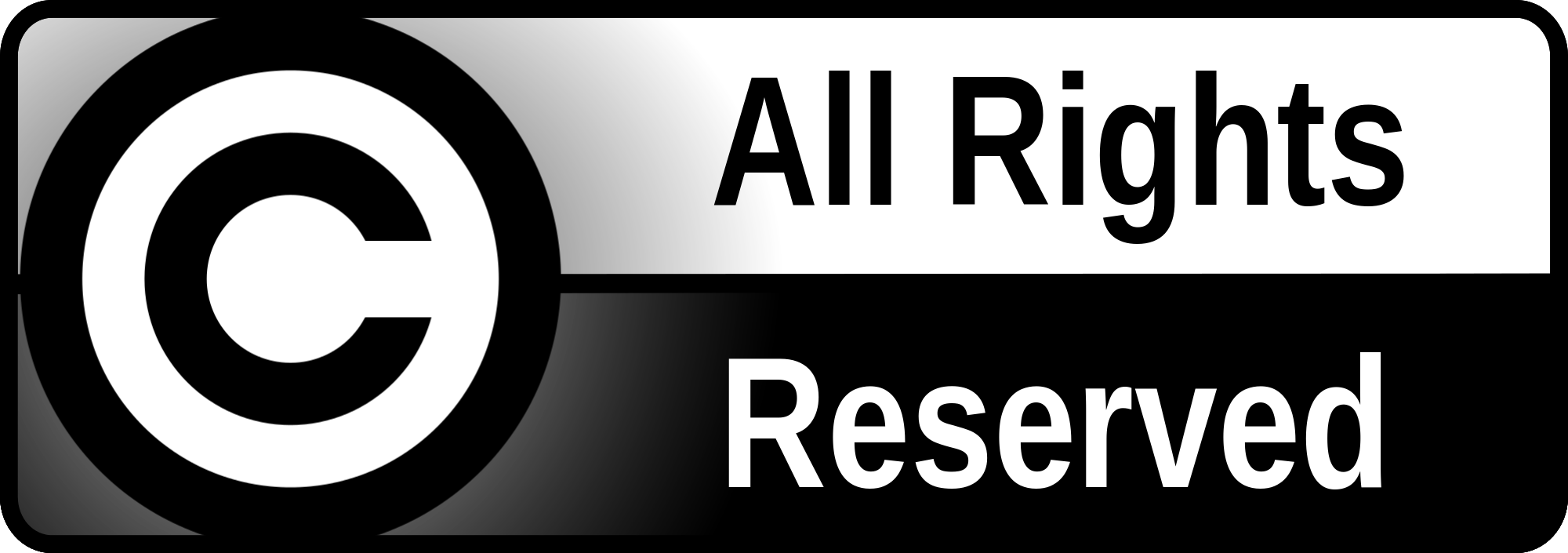
Метаподаци
Приказ свих података о документуАпстракт
The purpose of the present study was to model the effects of the concentration of Eudragit L 100 and compression pressure as the most important process and formulation variables on the in vitro release profile of aspirin from matrix tablets formulated with Eudragit L 100 as matrix substance and to optimize the formulation by artificial neural network. As model formulations, 10 kinds of aspirin matrix tablets were prepared. The amount of Eudragit L 100 and the compression pressure were selected as causal factors. In vitro dissolution time profiles at 4 different sampling times were chosen as responses. A set of release parameters and causal factors were used as tutorial data for the generalized regression neural network (GRNN) and analyzed using a computer. Observed results of drug release studies indicate that drug release rates vary widely between investigated formulations, with a range of 5 hours to more than 10 hours to complete dissolution. The GRNN model was optimized. The root me...an square value for the trained network was 1.12%, which indicated that the optimal GRNN model was reached. Applying the generalized distance function method, the optimal tablet formulation predicted by GRNN was with 5% of Eudragit L 100 and tablet hardness 60N. Calculated difference (f1 2.465) and similarity (f2 85.61) factors indicate that there is no difference between predicted and experimentally observed drug release profiles for the optimal formulation. This work illustrates the potential for an artificial neural network, GRNN, to assist in development of extended release dosage forms.
Кључне речи:
Artificial neural network / Aspirin / Controlled release / Eudragit L 100 / Matrix tabletsИзвор:
AAPS PharmSciTech, 2003, 4, 1Издавач:
- AAPS PharmSci Editorial Office
Институција/група
PharmacyTY - JOUR AU - Ibrić, Svetlana AU - Jovanović, M AU - Đurić, Zorica AU - Parojčić, Jelena AU - Petrović, Slobodan D. AU - Solomun, Ljiljana AU - Stupar, Biljana PY - 2003 UR - https://farfar.pharmacy.bg.ac.rs/handle/123456789/469 AB - The purpose of the present study was to model the effects of the concentration of Eudragit L 100 and compression pressure as the most important process and formulation variables on the in vitro release profile of aspirin from matrix tablets formulated with Eudragit L 100 as matrix substance and to optimize the formulation by artificial neural network. As model formulations, 10 kinds of aspirin matrix tablets were prepared. The amount of Eudragit L 100 and the compression pressure were selected as causal factors. In vitro dissolution time profiles at 4 different sampling times were chosen as responses. A set of release parameters and causal factors were used as tutorial data for the generalized regression neural network (GRNN) and analyzed using a computer. Observed results of drug release studies indicate that drug release rates vary widely between investigated formulations, with a range of 5 hours to more than 10 hours to complete dissolution. The GRNN model was optimized. The root mean square value for the trained network was 1.12%, which indicated that the optimal GRNN model was reached. Applying the generalized distance function method, the optimal tablet formulation predicted by GRNN was with 5% of Eudragit L 100 and tablet hardness 60N. Calculated difference (f1 2.465) and similarity (f2 85.61) factors indicate that there is no difference between predicted and experimentally observed drug release profiles for the optimal formulation. This work illustrates the potential for an artificial neural network, GRNN, to assist in development of extended release dosage forms. PB - AAPS PharmSci Editorial Office T2 - AAPS PharmSciTech T1 - Artificial neural networks in the modeling and optimization of aspirin extended release tablets with Eudragit L 100 as matrix substance VL - 4 IS - 1 DO - 10.1208/pt040109 ER -
@article{ author = "Ibrić, Svetlana and Jovanović, M and Đurić, Zorica and Parojčić, Jelena and Petrović, Slobodan D. and Solomun, Ljiljana and Stupar, Biljana", year = "2003", abstract = "The purpose of the present study was to model the effects of the concentration of Eudragit L 100 and compression pressure as the most important process and formulation variables on the in vitro release profile of aspirin from matrix tablets formulated with Eudragit L 100 as matrix substance and to optimize the formulation by artificial neural network. As model formulations, 10 kinds of aspirin matrix tablets were prepared. The amount of Eudragit L 100 and the compression pressure were selected as causal factors. In vitro dissolution time profiles at 4 different sampling times were chosen as responses. A set of release parameters and causal factors were used as tutorial data for the generalized regression neural network (GRNN) and analyzed using a computer. Observed results of drug release studies indicate that drug release rates vary widely between investigated formulations, with a range of 5 hours to more than 10 hours to complete dissolution. The GRNN model was optimized. The root mean square value for the trained network was 1.12%, which indicated that the optimal GRNN model was reached. Applying the generalized distance function method, the optimal tablet formulation predicted by GRNN was with 5% of Eudragit L 100 and tablet hardness 60N. Calculated difference (f1 2.465) and similarity (f2 85.61) factors indicate that there is no difference between predicted and experimentally observed drug release profiles for the optimal formulation. This work illustrates the potential for an artificial neural network, GRNN, to assist in development of extended release dosage forms.", publisher = "AAPS PharmSci Editorial Office", journal = "AAPS PharmSciTech", title = "Artificial neural networks in the modeling and optimization of aspirin extended release tablets with Eudragit L 100 as matrix substance", volume = "4", number = "1", doi = "10.1208/pt040109" }
Ibrić, S., Jovanović, M., Đurić, Z., Parojčić, J., Petrović, S. D., Solomun, L.,& Stupar, B.. (2003). Artificial neural networks in the modeling and optimization of aspirin extended release tablets with Eudragit L 100 as matrix substance. in AAPS PharmSciTech AAPS PharmSci Editorial Office., 4(1). https://doi.org/10.1208/pt040109
Ibrić S, Jovanović M, Đurić Z, Parojčić J, Petrović SD, Solomun L, Stupar B. Artificial neural networks in the modeling and optimization of aspirin extended release tablets with Eudragit L 100 as matrix substance. in AAPS PharmSciTech. 2003;4(1). doi:10.1208/pt040109 .
Ibrić, Svetlana, Jovanović, M, Đurić, Zorica, Parojčić, Jelena, Petrović, Slobodan D., Solomun, Ljiljana, Stupar, Biljana, "Artificial neural networks in the modeling and optimization of aspirin extended release tablets with Eudragit L 100 as matrix substance" in AAPS PharmSciTech, 4, no. 1 (2003), https://doi.org/10.1208/pt040109 . .